Dynamic Coupon Targeting Using Batch Deep Reinforcement Learning: An Application to Livestream Shopping
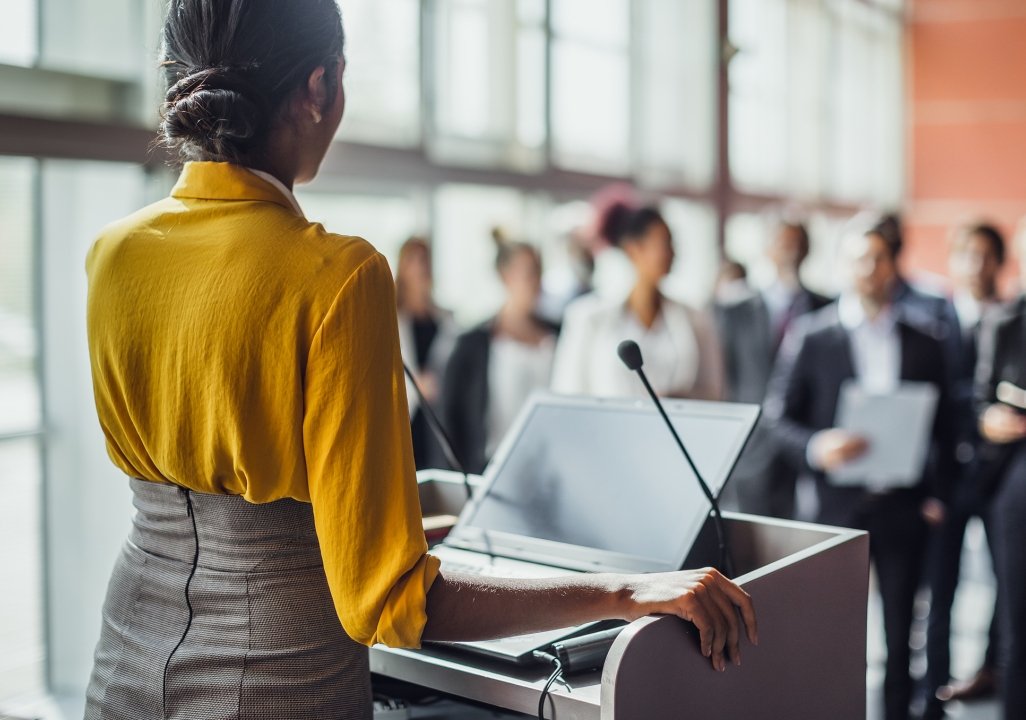
ABSTRACT
We present an empirical framework to create dynamic coupon targeting strategies for high-dimensional and high-frequency settings and test its performance using a large-scale field experiment. The framework captures consumers' intertemporal tradeoffs associated with dynamic pricing. It does not rely on functional form assumptions about consumers' decision-making processes. The model is estimated using batch deep reinforcement learning (BDRL), which relies on Q-learning, a model-free solution that can mitigate model bias. It leverages deep neural networks to represent the high-dimensional state space and alleviate the curse of dimensionality. The empirical application is in a multi-billion-dollar livestream shopping context. Our BDRL solution is twice as effective as static targeting policies, and 20% more effective than the model-based solution in increasing the platform's revenue. The comparative advantage of BDRL comes from better and automatically identifying when to target consumers (dynamics), and whom to target (heterogeneity), based on exceptionally rich nuanced differences in consumers and across time. For dynamics, we recommend price skimming. For heterogeneity, we recommend giving lower discounts when consumers visit more attractive hosts. Combining the two, we recommend increasing the coupon discount level at a faster rate for low spenders than for high spenders.