The Big Data Fallacy: A Consequence of the Difficulty of Identifying Omitted Variables
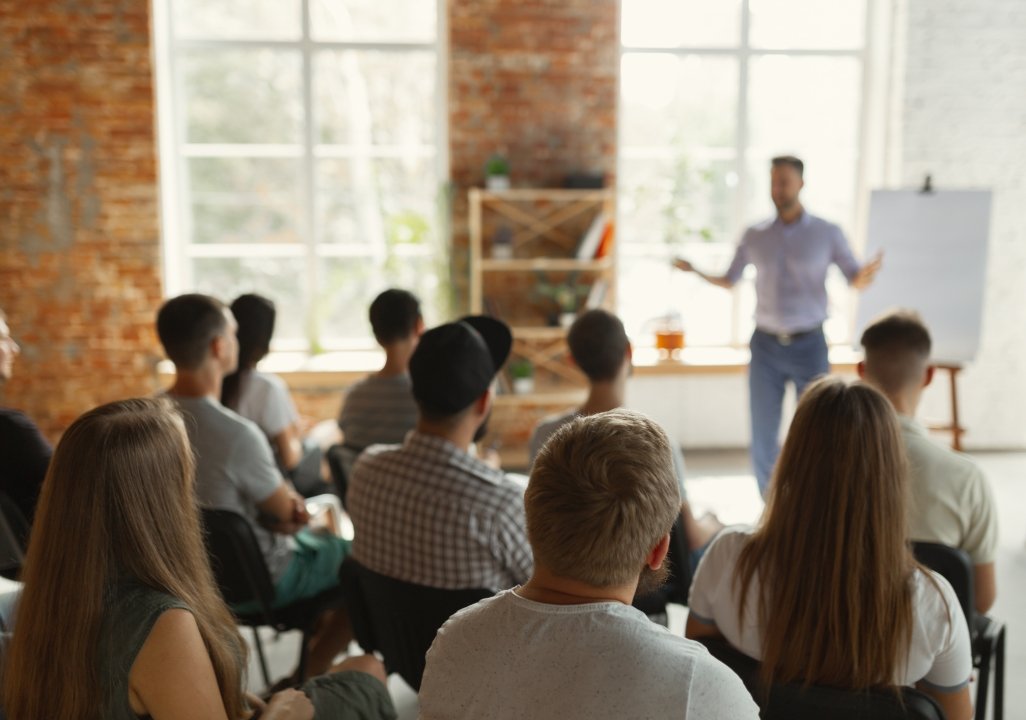
Abstract
Big data has revolutionized the way scientists, policy makers, and managers use empirics, with advocates of the big data revolution claiming that “with enough data, the numbers speak for themselves”. This is a dangerously misleading belief: since big data are typically drawn from observational sources rather than collected following the strict rules of random sampling or randomized experiments, they always feature some degree of sampling bias. When sample sizes are large, even the slightest sampling bias can produce much less accurate results than those obtained on small samples under random sampling/assignment. We demonstrate a behavioural consequence of this “Big Data Paradox”: decision-makers with varying levels of expertise believe that increasing data quantity necessarily increases data quality, and are hence more prone to erroneously interpret correlational evidence as indicative of causation when sample sizes are large. Training interventions teaching the difference between correlation and causation or the identification of omitted variables fail to alleviate this fallacy. Given these difficulties in debiasing the interpretation of evidence, we suggest strategies for the transparent communication of statistical results obtained on big data.