Artificial Intelligence in the Government: Responses to Failures and Social Impact
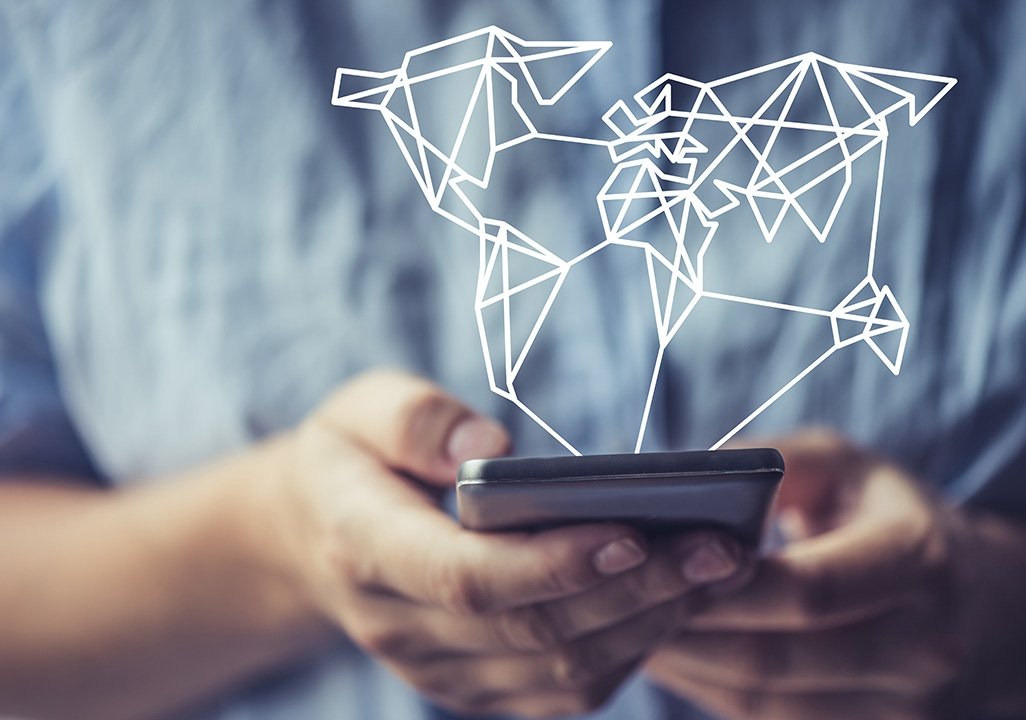
Abstract
Artificial Intelligence (AI) is pervading the government and transforming how public services are provided to people—from allocation of government benefits and privileges to enforcement of law and regulatory mandates, monitoring of risks to public health and safety, and provision of services to the public. Unfortunately, despite technological improvements and betterments in performance, AI systems are fallible and may commit errors. How do people respond when learning of AI’s failures? In twelve preregistered studies (N = 3,026) across a range of policy areas and diverse samples, we document a robust effect of algorithmic transference: algorithmic failures are generalized more broadly than human failures. Rather than reflecting generalized algorithm aversion, algorithmic transference is rooted in social categorization: it stems from how people perceive a group of non-human agents versus a group of humans—as out-groups characterized by greater homogeneity than in-groups of comparable humans. Because AIs are perceived as more homogeneous than people, failure information about one algorithm has higher inductive potential and is transferred to another algorithm at a higher rate than failure information about a person is transferred to another person. Assessing AI’s impact on consumers and societies, we show how the premature or mismanaged deployment of faulty AI technologies may engender algorithmic transference and undermine the very institutions that AI systems are meant to modernize.